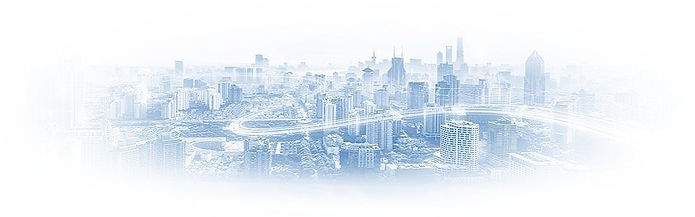
Assistant Professor
Structural Design & Mechanics
AI Lab for Design, Analysis, and Optimization
Faculty of Architecture & the Built Environment
Delft University of Technology
Engineered and natural environments are exposed to adverse operating conditions during their life-cycle, with
deterioration and hazards posing a constant threat to their reliability and resilience. Optimal decision-
making for such systems is concerned with controlling the extent and consequences of this exposure through
scheduling of efficient strategies, from preventive planning to post-disaster recovery, with the objective to minimize the involved socioeconomic and environmental risks. To be successful in this objective,
decision optimization needs to holistically alleviate multi-faceted complexities arising, among others,
by the curse of dimensionality related to large-scale multi-component systems; the curse of history
related to long-term sequential decisions; the model and data uncertainties; the operational constraints;
the presence of multiple agents and decision layers; and, finally, the sheer connection of physics-based
engineering models with the optimization process. This line of research studies solutions to the above
challenges through novel conceptual and computational frameworks within the contexts of stochastic optimization, systems reliability & control, and artificial intelligence. read more
Quantification of uncertainties in models and data constitutes the fundamental basis for quantifying risk, thus
being able to make informed engineering decisions. Towards this, uncertainties associated with chronic
deteriorating stressors (e.g. corrosion or fatigue) as well as with recurrent hazards (e.g. earthquakes
or hurricanes) need to be efficiently processed, either directly, based on data, or indirectly, propagated
through physics-based models. The goal is to efficiently estimate and infer certain probabilistic quan- tities of interest, but also to learn statistical structures that are able to forecast the dynamics of these
quantities in the absence of physics-based simulators. Probabilistic modeling in this regard has to
accommodate the presence of high dimensional feature and random variable spaces, as well as the
presence of correlations and dependencies at the spatial and temporal scales. This research is involved in
the study and development of such risk & reliability methods as these pertain to probabilistic performance-
based engineering, structural fragility analysis, Bayesian networks inference and learning, and supervised or unsupervised probabilistic machine learning. read more
​
Extreme events come with extreme loads, which force structural systems to their limit states. These states are often
manifested through large inelastic displacements and rotations that utterly defy linear predictions. Numerically
accurate and computationally efficient assessment of responses in materially and geometrically nonlinear
regimes is thus indispensable, especially in cases where statistical estimation and stochastic control are
the ultimate objectives. This line of research is involved in the study and development of computational
approaches, broadly pertaining to constitutive modeling of materials with coupled plasticity and
damage considerations, Bouc-Wen phenomenological hysteretic simulators, and structural element
formulations based on hybridized variational principles. In this direction, a major objective of this
research is to advance the joint platforms of computational structural mechanics and optimization,
as these relate to how nonlinear programming concepts can be integrated with the analysis process to
tame sources of computational complexity, and, reversely, to how highly nonlinear simulators can be integrated with optimization algorithms to drive dynamic design and structural intervention decisions. read more
Contact
Faculty of Architecture & the Built Environment
Delft University of Technology
Julianalaan 134, 2628 BL, Delft
email: c.andriotis [at] tudelft [dot] nl
Copyright © 2020-21 by C.P. Andriotis